Dark Data Utilization in Contact Center
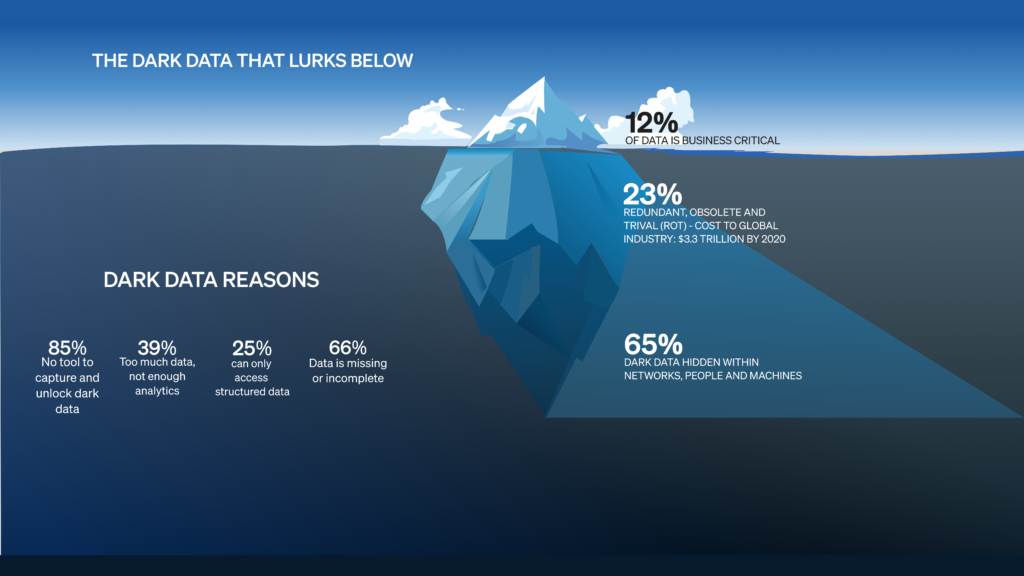
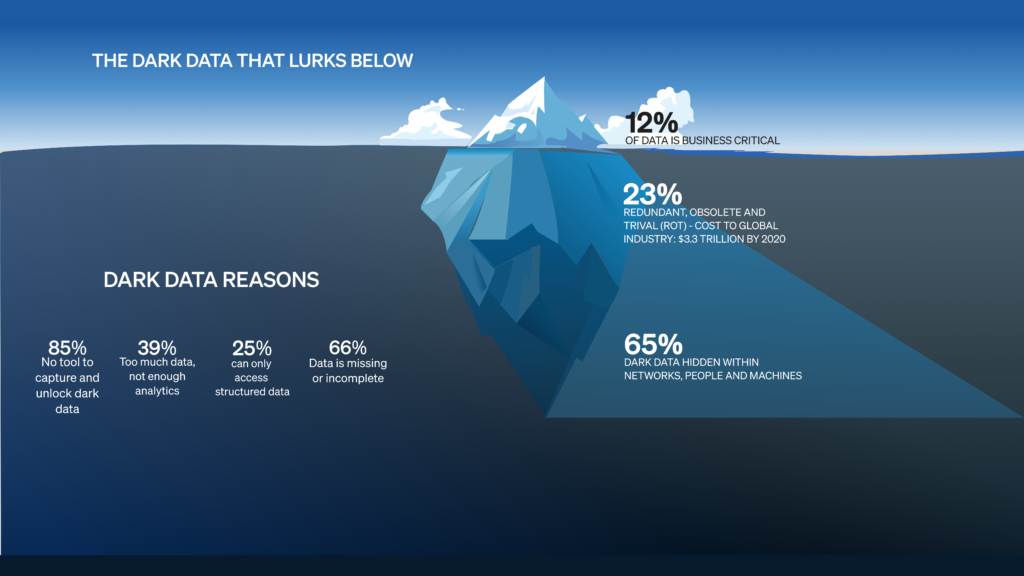
What is Dark data?
Dark data represents the latent pool of information gathered during routine business activities but often left untapped for further exploration or practical application. According to Splunk’s global survey of over 1,300 business and IT decision-makers, approximately 60% of organizations report that half or more of their data constitutes dark data, with one-third of respondents indicating that this unutilized data accounts for 75% or more of their overall data holdings. This accumulation of dark data stems from diverse sources, including abandoned projects, default data collection by applications or devices like IoT, and data rendered outdated due to insufficient analysis capabilities or storage limitations.
Contact centers generate vast amounts of unstructured data, constituting nearly 80% of the total data pool. This includes unutilized call recordings, abandoned chat sessions, and overlooked email interactions. Dark data significantly impacts operational efficiency, with approximately 60-70% of this unstructured data holding untapped potential for insights, indicating missed opportunities for improving service quality and operational efficiency. The presence of dark data affects customer experience, potentially leading to missed opportunities for personalization, targeted offerings, and enhanced issue resolution due to untapped customer insights.
Where does dark data come from?
Dark data encompasses information collected and stored by businesses but is rarely or never used for any business purpose. It primarily consists of unstructured data that accumulates from various sources, including:
- Log Files: Recordings of activities on company websites or apps, providing insights into customer behavior but often ignored due to bulk and complexity.
- Customer Behavior and Feedback: Valuable information about customer preferences and needs, is commonly collected but seldom analyzed by organizations.
- Social Media Interactions: Data from platforms like Facebook and Instagram, such as shares, likes, and comments, can enhance marketing strategies and offer deeper insights into customer behavior.
How to Utilize Dark Data for Customer Support?
Harnessing dark data for customer support involves strategic steps:
- Identify Sources: Recognize where dark data originates, such as social media, customer reviews, polls, and log files. Evaluate unutilized data your company collects to enhance customer service.
- Create an Analysis Plan: Develop a strategy to analyze and use the data. This may involve specialized tools or employing a team with data processing expertise. Define clear goals for data usage, like identifying customer behavior trends or refining customer service processes.
- Data Analysis and Insight Extraction: Employ data analysis techniques to uncover patterns or trends within the vast amount of data. Contextualize data collection to apply findings effectively within the company.
- Implement Insights: Translate extracted insights into action by modifying customer service strategies, enhancing support, or addressing common issues. Continuously monitor the impact of these changes and refine strategies based on ongoing data analysis.
Contact centers often accumulate vast amounts of data from various sources, including customer interactions, feedback, call recordings, emails, chat transcripts, and social media interactions. However, not all this data is structured or immediately usable. Techniques to recognize and categorize dark data might involve:
- Data Profiling and Discovery Tools: Utilizing specialized software and tools to scan and identify unstructured data within the contact center ecosystem. Data discovery tools have shown a 20-30% increase in uncovering previously unidentified dark data sources within enterprises according to Gartner. Forrester’s studies show that 80% of enterprise data is unstructured, making it challenging to identify and categorize without specialized tools.
- Content Analysis Algorithms: Employing algorithms that can sift through unstructured data, recognizing patterns or keywords that hint at valuable information. Algorithms utilizing natural language processing (NLP) can analyze customer feedback and sentiments, identifying trends and concerns. AI-powered content analysis tools can process vast volumes of unstructured text data, enabling the identification of valuable insights.
- Metadata Analysis: Extracting metadata to understand the nature, origin, and potential usefulness of unstructured data. Harvard Business Review reveals that Metadata analysis helps in understanding the context and relevance of unstructured data, contributing to a 25% increase in data accuracy. Deloitte’s survey states that proper metadata usage shows a reduction of up to 30% in misclassified data within enterprise databases.
Once dark data is identified, it’s crucial to distinguish it from datasets that hold actionable insights. Methodologies and tools to achieve this include:
- Data Quality Assessment: Employing data quality frameworks to assess the relevance, accuracy, and completeness of data sets. According to Gartner, data quality issues cost organizations an average of $15 million per year. Additionally, Experian reports that 40% of business initiatives fail to achieve their goals due to poor data quality.
- Data Tagging and Labelling: Implementing tagging systems to classify data as dark or potentially valuable, facilitating easier segregation. Effective data tagging, as stated by IDC, reduces data search time by 30-50% within enterprise systems. Moreover, proper labeling, as highlighted by McKinsey, enables a 25% improvement in the accuracy of segregating dark data from valuable datasets.
- Human Oversight and Expertise: Involving data analysts and subject matter experts to review and classify data based on contextual understanding. Involving subject matter experts in data classification processes improves accuracy by 20-30%, according to Data Science Central. MIT Sloan Management Review also notes that human oversight reduces misclassification rates by up to 15% in data segregation tasks.
Analytical Methods for Dark Data:
Extracting meaningful insights from unstructured data requires advanced analytical approaches such as:
- Text Mining and Natural Language Processing (NLP): Applying NLP algorithms to analyze text-based data like chat transcripts or customer feedback for sentiment analysis, topic modeling, and entity recognition. Towards Data Science reports that NLP-based sentiment analysis accuracy ranges from 70% to 90%, aiding in understanding customer emotions. Additionally, McKinsey states that text mining can increase the speed of insights extraction by up to 70% compared to manual analysis.
- Machine Learning Algorithms: Leveraging machine learning techniques like clustering or classification to categorize and derive patterns from unstructured data sets. According to the Harvard Business Review, machine learning models for dark data analysis achieve predictive accuracies of over 80% in customer behavior forecasting. Moreover, KDnuggets mentions that clustering algorithms can identify patterns in unstructured data with an accuracy increase of 25-30% compared to traditional methods.
- Predictive Analytics: Employing predictive models to forecast trends or customer behavior based on historical unstructured data patterns. Stanford University suggests that deep learning models improve the accuracy of image-based data analysis by 20-25% compared to conventional methods. Additionally, PwC notes that AI-driven analysis of unstructured data reduces processing time by up to 60%, expediting insights extraction.
Implementing machine learning and AI-based systems can aid in extracting insights from dark data by:
- Feature Engineering: Creating relevant features from unstructured data to make it suitable for machine learning models.
- Unsupervised Learning: Utilizing unsupervised learning algorithms like clustering to group similar data points within unstructured datasets.
- Deep Learning Approaches: Applying deep learning architectures like neural networks to process and derive insights from complex unstructured data formats like images or voice recordings.
Utilization Strategies:
Leveraging dark data for understanding customer behavior involves:
- Customer Segmentation: Using insights derived from dark data to segment customers based on preferences, behavior, or needs. As per McKinsey, segmentation based on dark data insights leads to a 25% increase in campaign conversion rates. Additionally, Forbes reports that personalized experiences from segmented data result in a 30% increase in customer retention.
- Personalization Strategies: Tailoring marketing campaigns or service offerings based on predictive models generated from dark data analysis. Accenture mentions that personalized recommendations driven by predictive models increase sales by 10-15%. Deloitte also highlights that dark data-informed personalization leads to a 20-25% improvement in customer satisfaction scores.
- Product Development Insights: Extracting insights from dark data to influence product/service enhancements or new offerings aligned with customer demands. For instance, McKinsey’s study showcases that 70% of successful product launches stem from understanding customer needs gathered through deep data analysis. Additionally, according to Harvard Business Review, leveraging dark data insights can lead to a 30% increase in the success rate of new product launches.
- Enhanced Routing and Issue Resolution: Leveraging insights to optimize call routing algorithms, ensuring customers are directed to the most suitable agents or departments based on their preferences and previous interactions, ultimately reducing resolution times. Research by Deloitte highlights a 25% decrease in average handling time when routing is aligned with customer preferences gathered from data analysis. Moreover, Zendesk reports a 20% increase in first-call resolutions by implementing tailored routing based on historical interaction patterns.
- Predictive Customer Needs: Employing predictive analytics derived from dark data to anticipate customer needs, allowing proactive service offerings and issue resolution before customers explicitly request assistance. Gartner’s research notes a 40% reduction in customer complaints when businesses anticipate needs before explicit customer requests. Furthermore, according to Forbes, organizations leveraging predictive analytics witness a 15% increase in customer satisfaction rates by addressing issues pre-emptively based on data-driven insights.
To ensure ethical use of dark data, implementing robust data governance frameworks includes:
- Compliance and Regulations: Ensuring that the utilization of dark data complies with privacy laws and industry regulations. According to GDPR Article 83, penalties could reach up to €20 million or 4% of the global annual turnover, whichever is higher.
- Ethical Guidelines: Establishing internal guidelines and policies for the ethical collection, storage, and utilization of dark data, emphasizing customer privacy and data security. Label Insight reports indicate that 91% of consumers tend to support brands they perceive as ethical.
- Data Security Measures: Implementing encryption, access controls, and data anonymization techniques to safeguard dark data from unauthorized access or breaches. As highlighted in IBM’s Cost of a Data Breach Report, the average cost of a data breach is $4.24 million.
By implementing these strategies and methodologies, contact centers can effectively identify, analyze, and ethically utilize dark data to derive actionable insights, improve customer experiences, and drive informed decision-making.